Projects
- Storage and processing of multi-word utterances
-
The ability to generate novel utterances compositionally is a hallmark property of human language. But speakers can also store and directly reuse utterances that have been heard before (e.g., I don’t know, salt and pepper). While traditional theories of syntax have focused on the role of generative knowledge, we argue that more is stored than is often assumed. In order to make these claims testable, we use computational models to formalize and quantify theories of the trade-off between composition and reuse. For example, is storage of multi-word utterances driven by raw frequency of the utterance, by the frequency of the utterance relative to the frequency of its component words, or by idiosyncrasy in its meaning? We then test the predictions of such models against experimental data.
Sample paper: Morgan, E. and Levy, R. (2016). Abstract knowledge versus direct experience in processing of binomial expressions. Cognition, 157, 382-402. - Language processing and language evolution
- How do speakers’ language processing biases shape a language across generations? What aspects of language structure (e.g., patterns of regularity versus idiosyncrasy) are attributable to synchronic language processing strategies? Combining theories of language processing with models of cultural evolution gives us a new way of testing how our theories of language processing and language evolution are mutually constraining.
Sample paper: Morgan E. and Levy R. (2016). Frequency-Dependent Regularization in Iterated Learning. In S.G. Roberts, C. Cuskley, L. McCrohon, L. Barceló-Coblijn, O. Fehér & T. Verhoef (eds.) The Evolution of Language: Proceedings of the 11th International Conference (EVOLANG11). - The role of expectations in human sentence processing
- Comprehenders use detailed statistical knowledge of a language in order to make predictions about upcoming linguistic material. We study how comprehenders make these predictions, how processing difficulty is jointly influenced by expectations and memory requirements, and how expectations can sometimes even override the linguistic input in cases of “noisy-channel” processing.
Sample paper: Delaney-Busch, N., Morgan, E., Lau, E., & Kuperberg, G. R. (2019). Neural evidence for Bayesian trial-by-trial adaptation on the N400 during semantic priming. Cognition, 187, 10–20. [pdf] - Bayesian models of semantics and pragmatics
- Modern statistical methods are allowing us to rethink the distinction between semantics and pragmatics, and to incorporate probabilistic expectations into our understanding of these domains. We are currently studying how people interpret quantifiers such as "many" or "several". The exact numeric meanings of these quantifiers are context-dependent: for example, “Melanie has many friends” versus “Melanie has many children” suggest different numbers of friends versus children. How do comprehenders use their knowledge of the world to put meaning to these different uses of the same quantifier? Methods such as Bayesian modeling and the Rational Speech Act model give us new ways of investigating these questions.
Sample paper: Reese, S., Jasbi, M. and Morgan, E. (2021, September). Bayesian Modeling of Quantifier Cardinal Reference Variability: The Case of English Few, Several, and Many. Oral presentation at Architectures and Mechanisms for Language Processing 2021. - Expectations in music
- Music is both similar to and different from language: both have hierarchical structure, but music lacks language’s propositional meaning. Nonetheless, expectations play a vital role in our enjoyment of music. Music sets up expectations for upcoming notes, rhythms, etc., and these expectations are strategically sometimes confirmed and sometimes violated in order to create an interesting listening experience. How do we form expectations about what notes will come next in a melody? To what extent do these expectations rely on domain-general statistical learning mechanisms, and to what extent do they rely on music- or auditory-specific principles (for example, the principles that allow us to separate out noises coming from different simultaneous auditory sources)? Applying theories and methods that have proven productive in language processing research often also yields insights into music cognition.
Sample paper: Morgan, E., Fogel, A., Nair, A., & Patel, A. D. (2019). Statistical learning and Gestalt-like principles predict melodic expectations. Cognition, 189, 23–34. [postprint] - Programming language comprehension
- Computer programming is an increasingly vital societal skill, which shares a lot of terminology with natural language (e.g., programming “languages”, coding “literacy”, etc.), sometimes leading educators and policy-makers to treat learning programming like learning a foreign language. But despite this intuitive link, we know relatively little about whether the cognitive processes that allow people to learn and use programming skills are the same as those underlying the learning and use of natural language. For example, do programmers rely on detailed statistical knowledge of patterns in code the same way that speakers of natural languages rely on detailed statistical language knowledge? We adapt methods from linguistics to ask these questions about programming, such as training statistical language models on code corpora and using these models to predict aspects of programmers’ comprehension.
Sample paper: Casalnuovo, C., Lee, K., Wang, H., Devanbu, P., & Morgan, E. (2020). Do Programmers Prefer Predictable Expressions in Code? Cognitive Science, 44(12). [postprint]
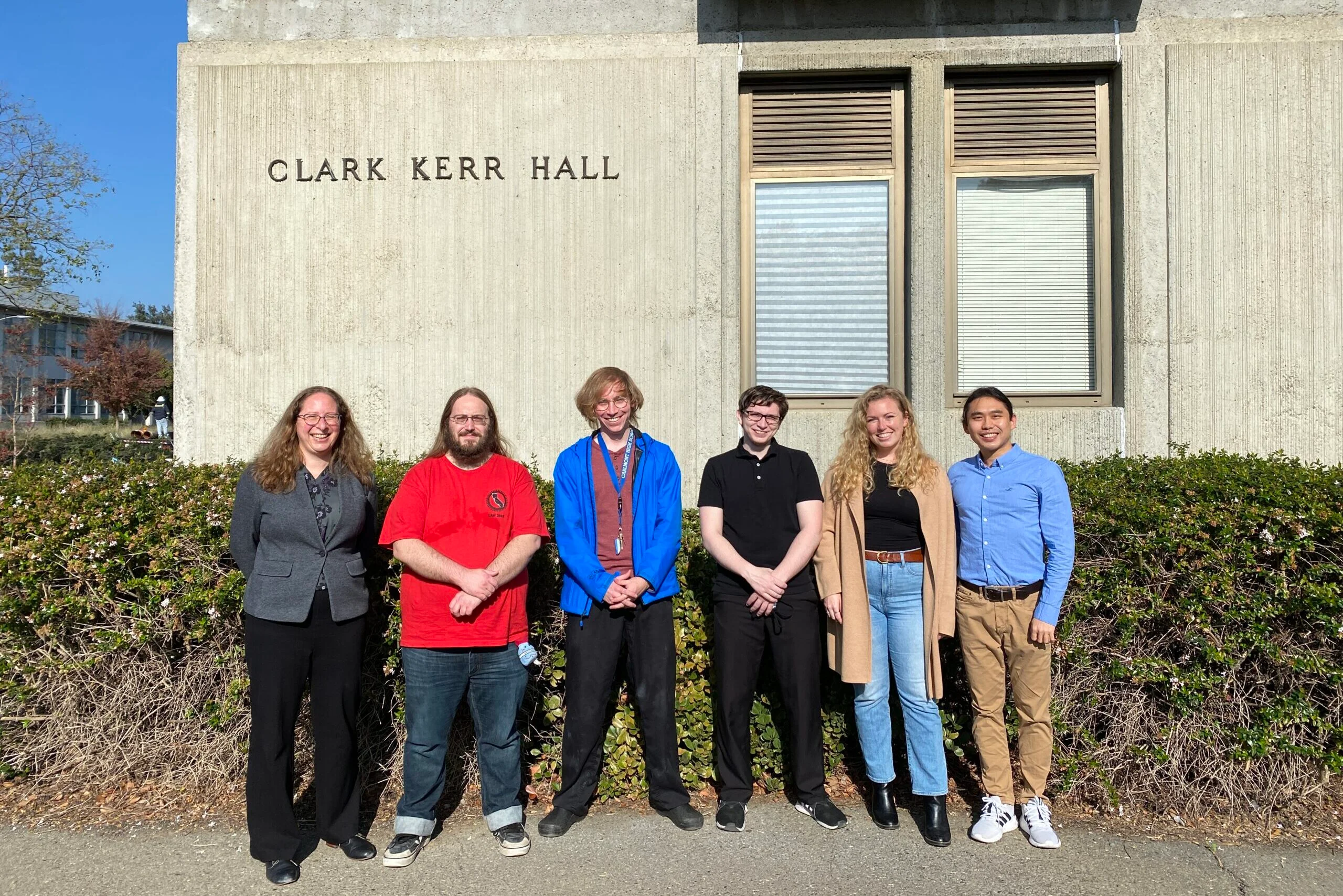
Selected Publications
- Brothers, T., Morgan, E., Yacovone, A., & Kuperberg, G. (2023). Multiple predictions during language comprehension: Friends, foes, or indifferent companions? Cognition, 241, 105602. https://doi.org/10.1016/j.cognition.2023.105602
- Jesse, K., Ahmed, T., Devanbu, P. & Morgan, E. (2023). Large Language Models and Simple, Stupid Bugs. To appear in: Proceedings of the 20th International Conference on Mining Software Repositories (MSR 2023). https://doi.org/10.48550/arXiv.2303.11455
- Chantavarin, S., Morgan, E. & Ferreira, F. (2022) Robust processing advantage for binomial phrases with variant conjunctions. Cognitive Science 46(9). https://doi.org/10.1111/cogs.13187
- Dodd, N., & Morgan, E. (2022). Expectations and Noisy-Channel Processing of Relative Clauses in Arabic. In Proceedings of the Annual Meeting of the Cognitive Science Society (Vol. 44, No. 44). https://escholarship.org/uc/item/51d7m5np
- Verosky, N. & Morgan, E. (2021). Pitches that Wire Together Fire Together: Scale Degree Associations Across Time Predict Melodic Expectations. Cognitive Science, 45(10). https://doi.org/10.1111/cogs.13037
- Fernandez Mira, P., Morgan, E., Sagae, K., Carando, A., Davidson, S., & Yamada, A. (2021). Lexical Diversity in an L2 Spanish Learner Corpus: The Effect of Topic-Related Variables. International Journal of Learner Corpus Research, 7:2. https://doi.org/10.1075/ijlcr.7.2
- Casalnuovo, C., Lee, K., Wang, H., Devanbu, P., & Morgan, E. (2020). Do Programmers Prefer Predictable Expressions in Code? Cognitive Science, 44(12). http://dx.doi.org/10.1111/cogs.12921
- Gonering, B. & Morgan, E. (2020). Uniform processing difficulty is a poor predictor of cross-linguistic word order frequency. In: Proceedings of the 24th Conference on Computational Natural Language Learning (CoNLL), pp. 245-255. https://www.aclweb.org/anthology/2020.conll-1.18
- Casalnuovo, C., Devanbu, P., & Morgan, E. (2020). Does Language Model Surprisal Measure Code Comprehension?. In: Proceedings of the 42nd Annual Conference of the Cognitive Science Society, pp. 564-570. https://cogsci.mindmodeling.org/2020/papers/0102/0102.pdf
- Liu, Z. & Morgan, E. (2020). Frequency-dependent Regularization in Constituent Ordering Preferences. In: Proceedings of the 42nd Annual Conference of the Cognitive Science Society, pp. 2990-2996. https://cogsci.mindmodeling.org/2020/papers/0750/0750.pdf
- Casalnuovo, C., Barr, E., Dash, S., Devanbu, P., & Morgan, E. (2020). A Theory of Dual Channel Constraints (NIER track). In: 2020 42nd International Conference on Software Engineering (ICSE). IEEE. https://dl.acm.org/doi/pdf/10.1145/3377816.3381720
- Morgan, E., Fogel, A., Nair, A., & Patel, A. D. (2019). Statistical learning and Gestalt-like principles predict melodic expectations. Cognition, 189, 23–34. http://doi.org/10.1016/j.cognition.2018.12.015 [postprint]
- Delaney-Busch, N., Morgan, E., Lau, E., & Kuperberg, G. R. (2019). Neural evidence for Bayesian trial-by-trial adaptation on the N400 during semantic priming. Cognition, 187, 10–20. http://doi.org/10.1016/j.cognition.2019.01.001 [pdf]
- Delaney-Busch, N., Morgan, E., Lau, E., & Kuperberg, G. (2017). Comprehenders Rationally Adapt Semantic Predictions to the Statistics of the Local Environment: a Bayesian Model of Trial-by-Trial N400 Amplitudes. In: Proceedings of the 39th Annual Conference of the Cognitive Science Society, pp. 283-288. [pdf]